Contents
From the Edge: Jeremy Glaser and Cliff Boro Talk Raising Money from Angels and VCs
AI: The Path of the Future or Industry Hype?
How to Leverage Privacy as a Key Competitive Advantage
Innovator Profile: Aphelion Path
Upcoming Events
In this episode of MinzEdge’s new From the Edge podcast, Attorney Jeremy Glaser talks to serial entrepreneur, investor, and Simplexity Holdings General Partner Cliff Boro about how entrepreneurs can best position themselves to raise money from angel investors and venture capitalists.
Cliff has extensive experience with raising money from angels and VCs: he has raised capital for over a dozen companies. Here, he relies on that experience to offer insights into the various processes through which entrepreneurs and start-ups can raise funds and the various questions that come up along the way. When it comes to raising capital, is all money created equal? How do you find VCs who will partner with you through the ups and downs that come with early stage start-ups? What should entrepreneurs focus on before they approach VCs or angel investors? Listen to this podcast for the answers to those questions and more.
Return to top
By Michael Renaud, Adam Rizk, and Jinnie Reed
Artificial intelligence (AI) — the science of teaching a machine how to “think” — has its roots in the 1950s. But until recently, it was considered a niche that was reserved for academics and government-sponsored research programs. And even today, AI’s overarching vision — building a machine that can exhibit the general intelligence of a human being — remains aspirational.
Nonetheless, companies are now racing to integrate AI into everything from self-driving cars to diagnosis of medical disease. What has driven AI back to the forefront is the emergence of sensors, big data, and computational horsepower. All of these factors have contributed to seismic advances in machine learning — teaching computers to identify patterns and make predictions by analyzing vast amounts of data rather than by following a specific program.
The most promising machine learning systems are powered by deep learning — a machine learning technique that uses big data to train computers to extract and evaluate complex hierarchical relationships from raw data (such as labelled images or speech) using progressive refinement through many sequential computational layers. These advanced systems are already all around us:
- Natural speech recognition & intelligent agents (e.g., Apple Siri, Microsoft Cortana, and Amazon Alexa)
- Photo recognition (e.g., Google images and Apple iCloud)
- Advanced Driver Assistance Systems (ADAS) installed in the 2018 Audi A8 and Cadillac CT6 to support “Level 3” autonomous driving (limited self-driving with human backup)
- Biometric security systems (e.g., Apple Face ID)
- Medical imaging (e.g., Enlitic’s tumor detection, Arterys’s blood flow quantification and visualization, and early detection of diabetic retinopathy)
- Manufacturing and robotics
In contrast to more traditional techniques, which apply a predetermined set of rules to distinguish between objects (e.g., a fox and a dog) based upon expected differences in their features (e.g., the shape of their ears or bushiness of their coats), deep-learning exploits big data to teach computers by example. The diagram below is illustrative of how this all works at a high level. The training and classification process occurs on a computer called a “neural net,” which is composed of a number of simple computing nodes called “neurons” organized in successive stages called “layers.” Each neuron responds to different features, and provides its result to neurons in a successive layer that process higher order features, and so on. During the classification “training” phase, the computer is fed with many examples (in this case, labeled images of different types of animals), and the neural net is taught how to classify images by iteratively adjusting the mathematical response of each individual neuron to its respective input feature until the error of predicting the labelled examples has been minimized. After the training phase, the computer can then classify unlabeled images. Mispredictions can be used to further refine the computer’s training; in other words, the computer is always learning.
Many companies and universities have open-sourced or otherwise contributed a substantial amount of deep-learning software to the public. Caffe (UC Berkeley), TensorFlow (Google), and Torch are among the popular software frameworks. Nonetheless, it is undoubtable that every major player has its own proprietary techniques.
AI-based innovations have been the subject of USPTO filings for many years now and, as illustrated below, companies operating in this space have traditionally executed on an aggressive IP protection strategy.
However, because these deep learning algorithms are perceived to be part of the software arts, the Alice decision and the ensuing uncertainty about the patentability of software have led to an abrupt decrease in U.S. filings as depicted below.
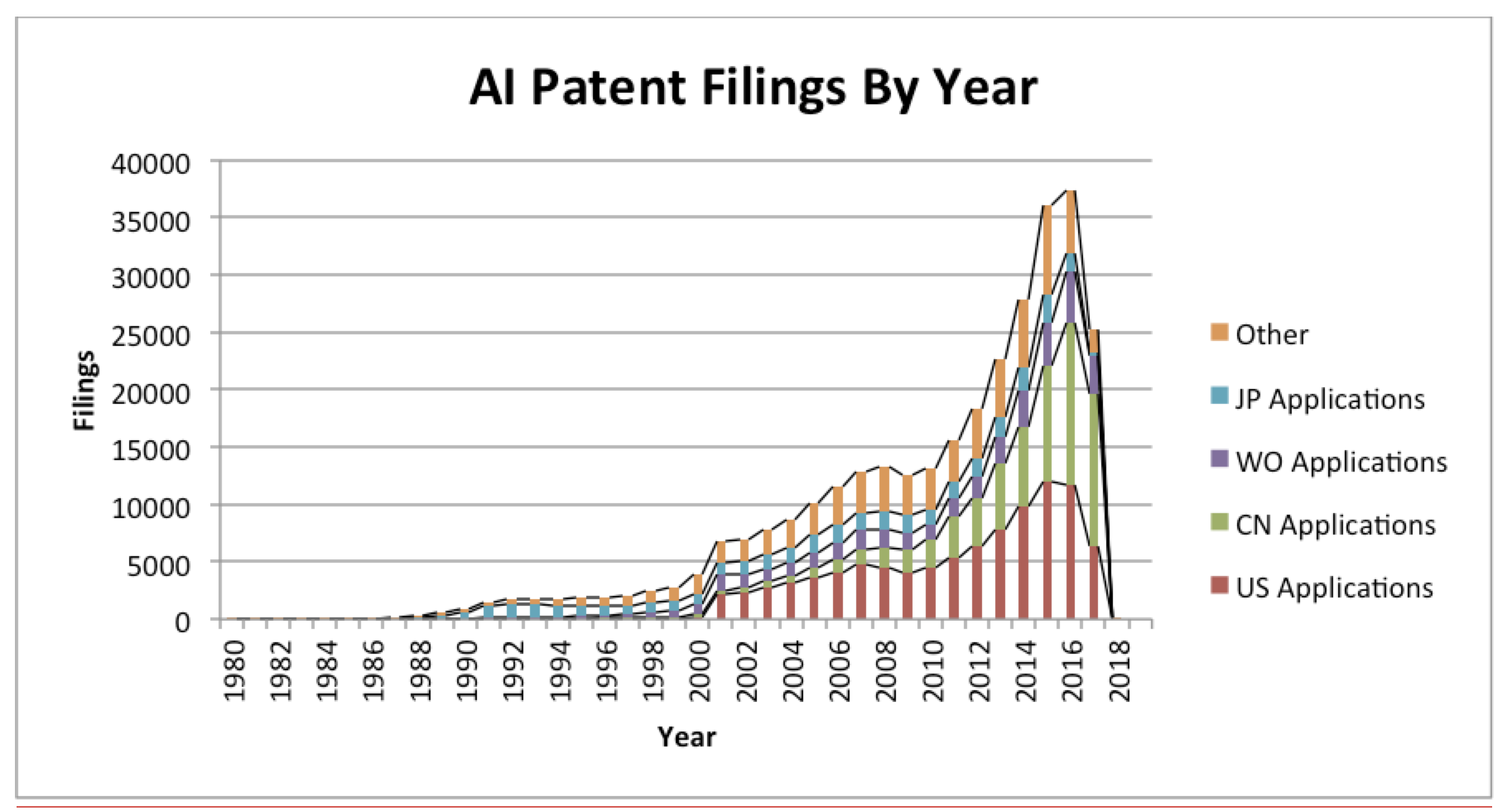 |
This signals that companies are not receiving sufficient counsel to confidently file patentable inventions in the AI space. Companies innovating in the area of AI should know that the Federal Circuit has now provided enough guidance to allow for patent applicants to direct claims to subject matter which will reliably survive Alice challenges. In short, AI claims that are crafted with enough care and precision won’t fail under section 101 Alice challenges if the patent discloses and claims a technical solution to a technical problem using a non-conventional combination of elements. The Mintz Levin team has written extensively about the line of Alice decisions and has deep experience securing and enforcing patent rights in view of these issues. Most recently in Finjan, Inc. v. Blue Coat Systems, Inc., 2018 U.S. App. LEXIS 601 (Fed. Cir. Jan. 10, 2018), the Federal Circuit confirmed its previous holdings from the Alice line of cases that “software-based innovations can make ‘non-abstract improvements to computer technology’ and be deemed patent-eligible subject matter[.]” Finjan, 2018 U.S. App. LEXIS 601 at *8 (quoting Enfish, LLC v. Microsoft Corp., 822 F.3d 1327, 1335-36 (Fed. Cir. 2016)). However, patentees need to continue to be mindful of providing sufficient disclosure of the way in which the claimed steps accomplish an advantageous result. Id. at *10-12.
The hardware platform that provides the computational layers is called a “neural net.” Graphics processing units or GPUs — computer hardware devices that were traditionally used to push pixels out to a screen to render 3D graphics — are a natural fit. Among other reasons, unlike central processing units or CPUs, which typically contain a limited number of complex computational resources, GPUs typically contain a network of hundreds or thousands of highly parallel single input multiple data (SIMD) processors that are organized in a way that is conducive to running deep learning algorithms.
While the goals of deep learning and 3D graphics processing are different, the underlying GPU hardware architectures that they use are similar if not identical to the architectures that were at issue in recent ITC investigations that the Mintz Levin team brought on behalf of its clients Graphics Properties Holdings (formerly Silicon Graphics), Advanced Silicon Technology, and Advanced Micro Devices (AMD). The key differentiator is that deep learning applications do not use all of the 3D graphics hardware. Rather, deep learning mainly makes use of the GPUs’ compute units. But GPUs often contain additional circuitry that are specialized to accelerate the process of 3D graphics rendering, such as “fixed-function” triangle setup units, scan converters, rasterizers, and texture units, and have limited utility to deep learning.
Over the past several years, a new class of hardware accelerators has emerged. These devices — coined “neural processing units” or NPUs — are similar to GPUs but do not have the added overhead of fixed function GPU circuitry and are optimized for AI in hardware to handle dot product math and matrix operations using lower precision numbers. However, unlike GPUs, which allocate compute resources on demand to service graphics and AI workloads to minimize idle time, NPUs are dedicated to just one task — AI processing. As a result, choosing the optimum hardware solution largely depends on the specific use case. Looking further into the future, the way in which machine learning algorithms (and the hardware architectures of the GPUs or NPUs that run these algorithms) evolve with time will also factor into the selection process.
Beyond refining the algorithms and the hardware, technology companies are competing to be first to market with meaningful new features that will truly differentiate them over their competitors. To be the winner requires much more than slapping a “powered by AI” label on the end-product. Among other things, it involves sifting through endless possibilities of how new sensor technologies, computational resources, and deep learning might be integrated together. Apple’s latest face unlock technology provides a prime example of this point. No less than six years ago (forever in the smart phone industry), Android-based devices were shipping with face unlock technology. Very much like Apple’s recently introduced Face ID, Android’s face unlock was powered by deep learning techniques. But the nearly universal consensus among Android users was that face unlock was nothing more than a marketing gimmick; it did not unlock well in low-lighting conditions, and in proper lighting conditions, it was vulnerable to being duped by a picture. Apple’s iPhone X caught the competition off guard. Having solved these problems by combining deep learning with more localized computational resources (for low latency) and a much more sophisticated set of sensors (including an infrared camera, a flood illuminator, a regular camera, and a dot projector), Apple transformed what was once a marketing gimmick into an actual selling point, sending every major company scurrying to develop and release “real” face unlock technology in its next-generation product.
In sum, while it is less than certain how far the technology will ultimately take us, it is clear that the field of AI is young, disruptive, and here to stay.
Return to top
BY BRIAN LAM AND CYNTHIA LAROSE
“Privacy by design” — while not a new concept — is certainly enjoying a new spot in the sunshine thanks to the European Union’s General Data Protection Regulation (“GDPR”) (43 days and counting…) and its codification of “privacy by design and default” in Article 25.
Privacy can also be a key differentiator and a competitive advantage. Read on for some points that can help drive your data privacy/data management program.
Currently, data breaches, as catalogued by the Identity Theft Resource Center (“ITRC”), a U.S. nonprofit set up to provide education and assistance with ID theft, found that data breaches had reached an all-time high in 2017. Analysis of the ITRC report showed that breaches were up 45% since 2016, with the business sector taking the hardest hit with 55% of breaches.
Further, existing data breaches may be even worse than thought or disclosed. For example, in addition to the initial breach Equifax revealed in September 2017, it has now described that additional types of information went missing. Uber has also struggled to confront its own data breach; Uber CISO John Flynn recently testified before the U.S. Senate Subcommittee on Consumer Protection, Product Safety, Insurance and Data Security that paying off the hackers was wrong, and that Uber should have provided notice to the affected public sooner.
Companies wondering how the public is dealing with this current landscape can rest assured that the public takes these issues seriously. A recent published report found that “69% of survey respondents said they would boycott a company known to lack adequate data protection.” Further, “more than half (55%) of respondents would avoid giving data to a company they know had been selling or misusing it before.”
Providing these data protections is no longer a nice-to-have. Consumers want to purchase from companies that value their privacy and security; failing to do so may cede a key competitive advantage.
A recent Harvard Business Review study examined the effects of data breaches on stock prices and found that data breaches could have significant effects on the stock price of a company experiencing the breach. Further, the study observed that competitors could be helped or harmed by the data breach depending on whether consumers viewed the competitor as either a better alternative, or somehow more risky in light of the existing data breach.
Underscoring actionable steps detailed here on Mintz Levin’s Privacy and Security Matters blog, the study went on to provide two basic strategies that when used by companies enabled the company “to protect or inoculate themselves from their own or a rival’s breach”:
- First, companies should explain in clear language how the company will be using and sharing customer data. This would include IP address and search history, for example.
- Second, companies should provide users control over their own data, giving the customer opportunities to opt out of certain practices, such as promotions.
The study found that customers did not punish breached companies that provided both transparency and control; instead, “empowered customers are more willing to share information and are more forgiving of data privacy breaches, remaining loyal after the fact.” Unfortunately, only around 10% of the Fortune 500 had implemented these two strategies in their data management practices. Empowering data subjects is a key component of the GDPR and may start to move the needle.
If you have any questions on how your organization can provide for and implement a transparent data management program that provides meaningful data controls to your customers, please do not hesitate to contact the team at Mintz Levin.
Return to top
Taking a start-up from idea to growth is the crucial step that can cause many founders to stumble and ultimately doom businesses to mediocrity. That’s why former operators at MakerBot, Zazzle, and Autonomous turned advisors to create the Aphelion Path Consulting Group.
Before starting their business in winter 2017 at a Brooklyn coffee shop, the founders of Aphelion Path had spent many years at start-ups but felt that working for just a few wasn’t enough. They wanted to take their learnings and experience to as many start-ups as possible to catch them before stumbling at that critical growth inflection point. Founding Aphelion Path as a start-up consulting group was the natural next move. At Aphelion Path, they take the best of what they’ve learned in start-up marketing, growth strategies, and organizational design and propagate it to many companies at once, increasing the impact of their knowledge and maximizing shared learning potential. In the short time that Aphelion Path has operated, they’ve already advised NYC and international start-ups Tylko, Umbrella, eponym, and more listed on their start-up consulting website.
Not to be “lumped together” with other consulting firms or individual consultants, the Aphelion Path team takes an open approach to the information and learnings they develop. Starting in the summer of 2018, they will be launching the T-48 program and the Mission Control blog (the Aphelion Path team is a fan of space/NASA). T-48 is a two-day weekend intensive where the Aphelion Path team embeds with a start-up to learn its systems and provide strategic recommendations by close of day on Sunday. It is offered completely free to any start-up that is approved for the program after an interview with the Aphelion Path team. Additionally, they are launching the Mission Control blog, a free resource of their training materials, strategic designs, and tactical execution documents, to help the start-ups that they cannot reach physically.
To apply for the first six slots of the T-48 program, email Aphelion Path here: [email protected].
Return to top
April 18: Empire Startups Fintech Conference 2018
April 23 – 24: Kayo Women’s Real Estate Investment Summit
April 30 – May 2: O’Reilly Artificial Intelligence
April 30 – May 4: Digital Content NewFronts
May 2: DLD New York
May 8 – 9: Techonomy
May 8 –10: WSJ’s Future of Everything Festival
May 9 –11: 99U Conference
May 10: TechDay New York
May 14 – 16: Consensus
May 22 – 24: DataDisrupt 2018
June 19 – 21: CB Insights Future of FinTech
June 20 – 21: Bloomberg Breakaway
July 11 – 12: Dash by Datadog
July 18: CNBC’s Delivering Alpha
April 12: Golden Seeds Office Hours
April 18 – 20: Cloud Foundry Summit
April 23: MIT Technology Review Presents: The Business of Blockchain
April 23 – 27: Boston Blockchain Week
April 25: The Ad Club CMO Breakfast with L.L. Bean
May 9: Retail Boost 2018
May 15 – 17: J.P. Morgan Global Technology, Media and Communications Conference
May 17: Small Business Expo 2018 - BOSTON
May 23: MIT Sloan CIO Symposium
June 17 – 20: LiveWorx Digital Transformation Conference
June 24 – 27: Identiverse
July 11 – 13: USENIX Annual Technical Conference
July 24: MITX Future of the Workplace
April 12 – 13: Big Data Innovation Summit
April 12 – 13: Internet of Things Summit
April 15 – 16: Forbes CIO Conference
April 16 – 17: AGC’s Information Security & Broader Technology Growth Conference
April 16 – 20: RSA Conference
April 25: Fighting Abuse @Scale
April 26: Founders’ Leadership Series with Robin Hayes, President and CEO, JetBlue
April 29 – May 2: Marketing Nation Summit
May 8 – 10: Red Hat Summit
May 9 – 10: TRAIN AI
May 14 – 15: PG Connects
May 14 – 16: Apttus Accelerate
May 22: Slack Spec Developer Conference
May 22 – 24: Pure Accelerate
May 31 – June 1: QueryCon
June 4 – 6: Spark+AI Summit
June 12 – 15: DockerCon
June 13 – 14: Girls in Tech Catalyst Conference
June 16 – 19: Blockchain Economic Forum
June 20 – 21: DocuSign Momentum
June 24 – 28: Design Automation Conference
June 28: 500 Startups Demo Day
July 11 – 12: Wearable Technologies Conference
July 24 – 26: Google Cloud Next
April 19: UCSD Research Expo 2018
April 26: Xconomy Forum: Big Data Meets Big Biology
April 30 – May 2: Gartner Tech Growth & Innovation Conference
May 17 – 19: 4th Annual Conference on Business Research and Management Practices in Global Environments
June 25 – 29: San Diego Startup Week
June 28 – 29: 4th Annual SD Venture Group VC Road Trip
June 29: 6th Annual VC Regatta
April 17: ATARC Federal Emerging Technology Summit
April 19: Constellation Washington DC - Explore
April 24: Pitch Expo and Startup Gauntlet
April 26: The Power of Women from Wall Street to Entrepreneurship: Sallie Krawcheck
April 26: Mid-Atlantic Marketing Summit: Washington 2018
May 16 – 17: VCs-to-DC
May 16: Financing Your Business with Local Resources
Return to top
Daniel I. DeWolf (Editor)
Member, New York
212.692.6223
[email protected]
Samuel Effron (Editor)
Member, New York
212.692.6810
[email protected]
Susan J. Cohen
Member, Boston
617.348.4468
[email protected]
Sebastian E. Lucier
Member, San Diego
858.314.1519
[email protected]
Cliff Silverman
Associate, New York
212.692.6723
[email protected]
Talia Primor
Associate, New York
212.692.6740
[email protected]
|
Jeremy D. Glaser
Member, San Diego
858.314.1515
[email protected]
Dean Zioze
Member, Boston
617.348.4795
[email protected]
Adam C. Lenain
Member, San Diego
858.314.1878
[email protected]
Lewis J. Geffen
Member, Boston
617.348.1834
[email protected]
Kristin Gerber
Associate, Boston
617.348.3043
[email protected]
Kaitlin Fox
Associate, New York
212.692.6759
[email protected]
|
Marc D. Mantell
Member, Boston
617.348.3058
[email protected]
Sahir Surmeli
Member, Boston
617.348.3013
[email protected]
Gregory Chin
Member, San Francisco
415.432.6017
[email protected]
Yilei He
Associate, New York
212.692.6232
[email protected]
Rachel Gholston
Associate, New York
212.692.6244
[email protected]
|
|