EnforceMintz — Artificial Intelligence and False Claims Act Enforcement
Like most industries, the health care sector is grappling with the uses of artificial intelligence (AI) and what AI means for the future. At the same time, many health care companies already have integrated algorithms and AI applications into their service offerings and thus should be considering compliance risks associated with those tools.
While there has been limited AI-related enforcement to date, we can predict how AI-related enforcement may develop based on previous technology-related enforcement. We likewise can anticipate how relators and enforcement agencies might use AI to detect potential fraud and develop allegations based on how technology has already been used for these purposes.[1] The bottom line is that the use of AI to identify targets for enforcement, as well as AI-related allegations, likely will continue to evolve as rapidly as the technology itself.
Use of AI in Processing or Submitting Information to Federal Health Care Programs Without Appropriate Human Oversight
Enforcement actions involving companies that use algorithms and other technologies to process, review, and submit claims have been ongoing for years, and we expect to see similar enforcement actions taken against companies using AI tools. For example, health plans using technology to review claims or to assess patient medical records for evidence of certain diagnoses already have been subject to enforcement. Typically, the fraud alleged relates to the use of algorithms or applications for these functions without appropriate human involvement, oversight, or both. For example, in 2021, DOJ intervened in several False Claims Act (FCA) qui tam lawsuits alleging in part that a health plan used a natural language processing (NLP) algorithm to gather and submit inaccurate diagnosis codes for its Medicare Advantage (“MA”) enrollees to receive higher reimbursements. As discussed here, MA plans generally receive higher payments to cover the cost of care for members who have more severe or complex health conditions (reflected in diagnosis codes).
The lawsuits against this health plan alleged, in part, that the plan created its own NLP audit program to find new diagnosis codes to submit to CMS, and that the NLP program used an algorithm to search electronic medical records for words indicating that a patient had certain diagnoses. While MA plans commonly use NLP tools for various purposes, the health plan at issue here was accused of submitting false claims to CMS for risk adjustment because it knew that some of the claims prepared using its NLP tool contained errors that the plan failed to detect or correct, in some circumstances. For example, the government accused the health plan of submitting diagnoses to CMS for conditions for which the relevant patients were not being treated. As of the date of publication, these matters are still in litigation.
These types of allegations against MA plans, and health plans more generally, are not new. But given the many existing and emerging uses of AI, we expect that enforcement will focus on the identification, collection, and submission of allegedly inaccurate data to federal health care programs, including MA.
Use of AI to Perform Services that Human Providers Should Perform
Another area where the use of AI could raise potential FCA risks is where recommendations or suggestions made by an AI tool run afoul of federal health care program coverage requirements. For example, for diagnostic X-rays and laboratory tests (among other diagnostic tests) to be considered “reasonable and necessary” for purposes of Medicare coverage, those services “must be ordered by the physician who is treating the beneficiary, that is, the physician who furnishes a consultation or treats a beneficiary for a specific medical problem and who uses the results in the management of the beneficiary’s specific medical problem.”[2] AI tools could potentially create risk where providers use those tools to order items or services without appropriate provider involvement and oversight. For example, if AI were used to select a test, and that order did not meet coverage requirements, this activity could give rise to a false claim.
We already have seen government enforcement actions that raised similar issues. For example, we previously covered a January 2020 settlement between DOJ and a developer of cloud-based electronic health records (EHR) systems. The company paid $145 million to resolve criminal and civil investigations and entered into a deferred prosecution agreement. DOJ alleged that the EHR company violated the Anti-Kickback Statute (AKS) by receiving kickbacks from an opioid company to influence physicians to prescribe certain opioid pain medications by sending clinical decision support (CDS) alerts to those physicians through the EHR system.
More specifically, the government alleged that the EHR company allowed “pharmaceutical companies to participate in designing the CDS alert, including selecting the guidelines used to develop the alerts, setting the criteria that would determine when a health care provider received an alert, and in some cases, even drafting the language used in the alert itself. [Moreover, the] CDS alerts that [the EHR company] agreed to implement did not always reflect accepted medical standards.” DOJ described this conduct as “abhorrent,” noting that the company had “allow[ed] an opioid company to inject itself in the sacred doctor-patient relationship so that it could peddle even more of its highly addictive and dangerous opioids.”
While this case did not involve allegations related to AI, it does provide some insight into how the government might approach conduct that it perceives as comparable. In particular, the government might take issue with AI uses that it views as improperly inserting technology into the doctor-patient relationship and using AI to make decisions or recommendations that are inappropriate or inconsistent with accepted medical standards.[3]
Use of AI Could Raise a Host of Other Potential Regulatory Issues
Our FDA colleagues have previously covered a variety of FDA regulatory issues that may arise with respect to the use of AI in health care (you can access some of their posts here, here, and here). But, in addition to the risk areas we note above, use of AI that does not have required FDA approval or clearance, if applicable, likewise raises risk under the FCA. For example, the use of unapproved medical devices — or the use of devices for unapproved purposes — could run afoul of the FCA.
Relatedly, if an AI tool does not perform as expected or “learns” or evolves in such a way that the tool is no longer safe or effective or no longer performs an approved purpose, these changes could raise a spectrum of legal risks, including an allegation that the services rendered with that tool are “worthless” and thus claims for those services raise FCA risks.
AI Could Offer Tools for Whistleblowing and Enforcement Purposes
Beyond the many benefits (and risks) that AI could create for health care providers and innovators, AI likewise can offer many tools for relators and enforcement officials. For example, generative AI tools could be used to analyze reams of company records and draft legal complaints. Or AI tools could be used to compare and analyze information and data across massive databases and other online sources. Pre-AI, this type of analysis would have required the dedication of substantial time and human resources. With AI, this type of analysis is relatively straightforward and efficient.
In fact, we already are seeing such AI strategies in use, and we likewise are observing the interesting legal challenges they raise. For example, in cases where relators have used AI tools to compare information from a variety of publicly available internet sources and databases to establish the allegations in their complaint, defendants have moved to dismiss on the grounds that the complaint is barred by the public disclosure bar. Courts are now having to grapple with whether the sources that the relators relied upon qualify as a source of “public disclosure.”
In at least one state court case, the court found that the relator’s complaint was not barred because the websites that the relator relied upon did not meet the definition of “news media,” and thus, the relator’s allegations were not “public disclosures” under the state’s law. We expect to see many more cases of this nature in the future, and they will undoubtedly have an impact on FCA case law at both the state and federal levels.
Much like the technology itself, AI-related enforcement is still in its early days — and this piece only touches on a few potential ways that we may see AI usage appear in FCA enforcement. As is the case with so many enforcement areas, we will certainly continue to monitor these developments and report back as we learn more.
Endnotes
[1] For example, the Department of Justice has used data mining for many years to detect potential false claims or questionable billing practices. DOJ’s Heath Care Fraud Unit describes itself as “a leader in using advanced data analytics and algorithmic methods to identify newly emerging health care fraud schemes and to target the most egregious fraudsters. The Unit’s team of dedicated data analysts work with prosecutors to identify, investigate, and prosecute cases using data analytics. This novel approach has led to some of the Fraud Section’s largest cases and initiatives.” See US Department of Justice Criminal Division Health Care Fraud Unit website: https://www.justice.gov/criminal/criminal-fraud/health-care-fraud-unit.
[2] 42 C.F.R. § 410.32(a).
[3] Relatedly, on January 8, 2024, the Department of Health and Human Services and Office of the National Coordinator for Health Information Technology (ONC) published the “Health Data, Technology, and Interoperability: Certification Program Updates, Algorithm Transparency, and Information Sharing (HTI-1)” Final Rule. The HTI-1 Final Rule featured several key updates to the ONC Health IT Certification Program (Program), including new transparency requirements for certain AI components and predictive algorithms that are built into certified health IT. We covered this Final Rule in more detail in this post.
Authors
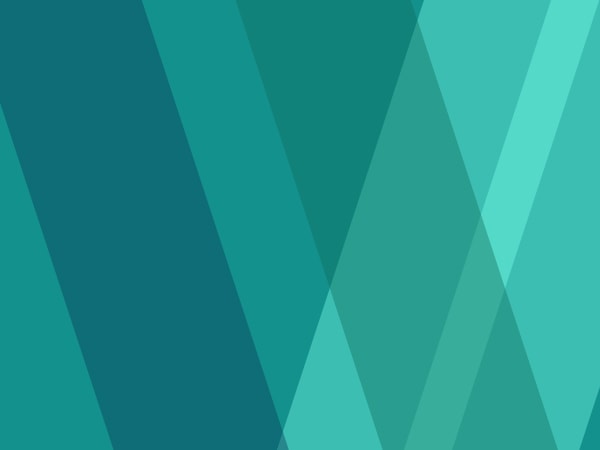