DC Circuit Court Rules AI Cannot be Author of Copyrighted Work, and NIST Finalizes AI Report — AI: The Washington Report
- On March 18, the US Court of Appeals for the DC Circuit ruled that an AI model cannot be the author of copyrighted material under existing copyright law. The court affirmed the US Copyright Office’s long-standing human authorship requirement for copyright protections.
- The decision comes as other copyright questions raised by AI advancements are also being litigated in federal courts. As Congress has not moved to modify copyright laws for AI, courts may play an increasingly large role in clarifying how existing copyright laws apply to AI and AI-generated works.
- In a separate matter, on March 24, the National Institute of Standards and Technology (NIST) published its final report on Adversarial Machine Learning, which includes a taxonomy of attacks against AI systems. The report provides voluntary guidance for mitigating these attacks.
On March 18, the US Court of Appeals for the DC Circuit ruled that an AI model cannot be the author of copyrighted material. The court affirmed the US Copyright Office’s long-standing human authorship requirement for copyright protections. The decision comes as other copyright questions raised by AI advancements are also being litigated in federal courts, which suggests that courts may play an increasingly large role in clarifying how existing copyright laws apply to AI and AI-generated works
In other AI news, on March 24, the National Institute of Standards and Technology (NIST) published its final report on Adversarial Machine Learning, which includes a taxonomy of attacks against AI systems. The report also provides voluntary guidance for the AI and cybersecurity communities to mitigate these attacks.
DC Circuit Court Affirms AI Cannot be Author of Copyrighted Work
On March 18, the D.C. Circuit Court ruled that an AI system by itself cannot be the author of copyrighted material, affirming the human-authorship requirement for copyrightability. “The text of multiple provisions of the [Copyright Act] indicates that authors must be humans, not machines,” according to the court’s decision.
The case involved a computer scientist who created an artwork using AI and filed a copyright application for that artwork. The computer scientist listed an AI model as the artwork’s author on the application and wrote that the artwork was “created autonomously by machine.” The US Copyright Office denied the application because “a human being did not create the work.”
In 2023, the US District Court for the District of Columbia upheld the Copyright Office’s rejection of the computer scientist’s application. The district court concluded that “human authorship is a bedrock requirement of copyright.”
The DC Circuit Court upheld the lower court’s decision based on the Copyright Act’s provisions, which “make sense only if an author is a human being,” according to the opinion. The Act requires signatures for copyright transfers, but “machines lack signatures.” The Act limits the copyright’s duration to the author’s lifespan, but “machines do not have lives.” And the Act protects authors regardless of their “nationality or domicile,” but “machines do not have domiciles, nor do they have a national identity.” “All of these statutory provisions collectively identify an ‘author’ as a human being,” according to the court.
The DC Circuit Court’s decision confirms the US Copyright Office’s view that human authorship is necessary for copyrightability. According to the Office’s 2023 guidance, “it is well-established that copyright can protect only material that is the product of human creativity. Most fundamentally, the term ‘author,’ which is used in both the Constitution and the Copyright Act, excludes non-humans.” The Office reaffirmed the human-authorship requirements in part two of its Report on AI Copyrightability in February 2025, which we wrote about.
The DC Circuit emphasized that it was up to Congress to decide whether the Copyright Act should be updated to one day allow AI-generated works to be copyrightable. The court argued that “even if the human authorship requirement were at some point to stymie the creation of original work, that would be a policy argument for Congress to address.”
This year, courts may also confront the question of the use of copyrighted materials to train AI models. As we’ve written about, lawsuits brought by the creative industry have challenged AI companies’ use of copyrighted materials and information to train their AI models. The US Copyright Office is also working on a forthcoming report addressing the same question.
NIST Finalizes Report on AI Attacks and Mitigation Strategies
On March 24, NIST published its final report on Adversarial Machine Learning: A Taxonomy and Terminology of Attacks and Mitigations. “The statistical, data-based nature of ML systems,” according to the report, “opens up new potential vectors for attacks against these systems’ security, privacy, and safety, beyond the threats faced by traditional software systems.”
The report includes a standardized taxonomy of attacks for two different types of AI systems – Predictive AI (PredAI) and Generative AI (GenAI) systems – and voluntary mitigation strategies for the AI and security communities to consider adopting.
For PredAI systems, the report identifies three of the most widely studied attacks and mitigation strategies:
- Evasion attacks involve manipulating input data to make incorrect output data. Mitigation strategies include access controls, data sanitization and validation methods, and dataset provenance and integrity attestation mechanisms.
- Poisoning involves attacks that corrupt the data sets used to train AI models. Poisoning mitigations include training data sanitization, trigger reconstruction, and model sanitization and inspection.
- Privacy Attacks are malicious attempts to compromise user data by exploiting vulnerabilities in AI systems. The main defense against privacy attacks is differential privacy (DP) mechanisms, which aim to create a “bound on how much an attacker with access to the algorithm output can learn about each individual record in the data set.” DP mechanisms include the Gaussian mechanism, the Laplace mechanism, and the Exponential mechanism, among others.
The report also identifies the three main attacks against GenAI systems and mitigation strategies:
- Poisoning for GenAI systems also involves attacks that corrupt the data sets that the AI system trains with. “GenAI poisoning mitigations largely overlap with PredAI poisoning mitigations,” according to the report. Mitigation strategies include verifying web downloads before entering new data into the data set and data filtering to remove poisoned samples.
- Direct Prompting Attacks feed AI models prompts that are designed to manipulate the model’s outputs. Mitigations include adversarial training methods, evaluation of the AI system for vulnerabilities, and numerous interventions during deployment, including prompt instruction techniques, harmful interaction detection, and prompt stealing detection.
- Indirect Prompt Injection Attacks covertly insert malicious information or instructions into the data set of an AI system. Mitigation involves training techniques, including fine-tuning task-specific models; detection schemes that detect indirect prompt injection; and input processing methods, including “filtering out instructions from third-party data sources” and “instructing models to disregard instructions in untrusted data.”
The report updates NIST’s 2023 Adversarial Machine Learning: A Taxonomy and Terminology of Attacks and Mitigations report, which was published in January 2024. The latest version of the report includes updated mitigation strategies and more in-depth discussion about potential attacks at each stage of the AI system lifecycle.
We will continue to monitor, analyze, and issue reports on developments about the Trump administration’s approach to and policies for AI, as well as court decisions involving AI.

Trump Administration Receives 8,755 Comments for AI Action Plan — AI: The Washington Report
March 21, 2025| Article|
Authors
Bruce D. Sokler
Member / Co-chair, Antitrust Practice
Alexander Hecht
ML Strategies - Executive Vice President & Director of Operations
Christian Tamotsu Fjeld
Senior Vice President
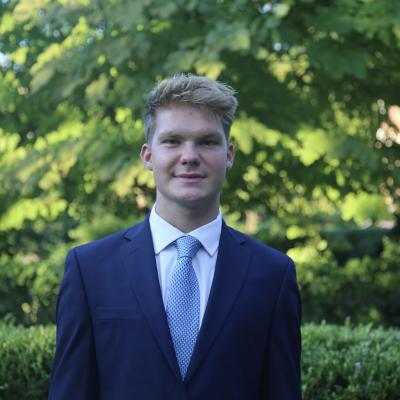